Podcasts
DCG Webinars
DCG Speaking Events

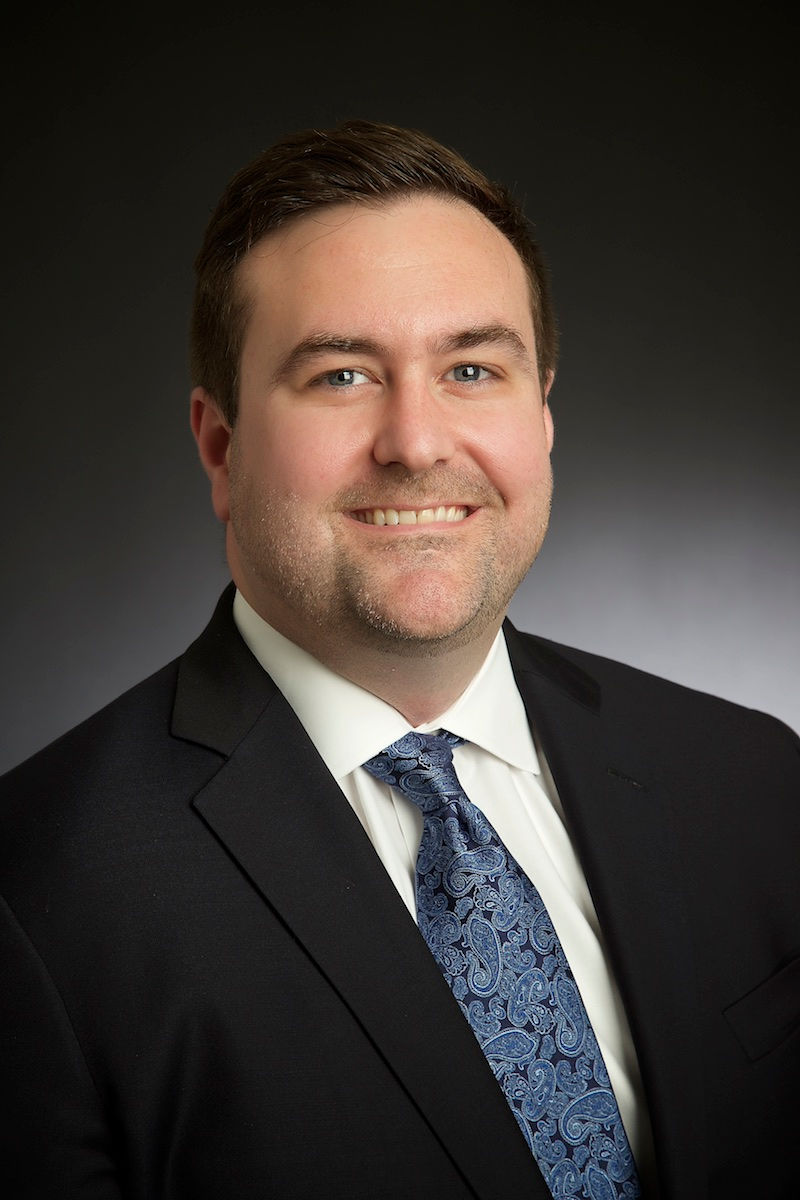
Chase Ogden
Quantitative Consultant
Darling Consulting Group
As a consultant with DCG’s Quantitative Risk Analysis and Strategy team, Chase brings over a decade of programming and modeling expertise. Chase provides a unique perspective of the entire analytics lifecycle, having served in a variety of roles from model developer to senior leader of enterprise-wide, cross functional analytics implementations.
As a practitioner at large and mid-sized financial institutions, Chase has experience in a wide array of modeling approaches, applications, and techniques, including: asset-liability models, pricing and profitability, capital models, credit risk and allowance models, operational risk models, deposit studies, prepayment models, branch site analytics, associate goals and incentives, customer attrition models, householding algorithms, and next-most-likely product association.
Chase is a graduate of the University of Mississippi and holds Master’s degrees in International Commerce Policy and Applied Statistics from George Mason University and the University of Alabama, respectively. A teacher at heart, Chase frequents as an adjunct instructor of mathematics and statistics.
Practitioners have widely observed a disconnect between macroeconomically modeled credit stress and the COVID reality. Of course, there are myriad reasons why macroeconomic forces cannot solely account for the variability in outcomes. Sustained and unprecedented government intervention was the primary contributor, offsetting default through direct payments to consumers, loans to many businesses, and encouraging deferrals to affected borrowers. While these issues were not directly considered in credit stress models, management teams quickly learned, by necessity, how to reduce loss forecasts due to prevailing conditions.
However, as we move sufficiently past the most turbulent COVID-related period, model updates are being challenged with troublesome new in-sample data. What were wildly changing macroeconomic factors driving forecast volatility during the recession are now outside the norm actual values. In order to roll models forward to incorporate new data, model owners are considering how to account for the counterintuitive relationship between their existing predictors and actual losses.
This typically involves understanding and addressing technical challenges such as outliers, influence, leverage, smoothing, and overrides.